December 18, 2023
Related articles
Why AI Governance Requires Data Governance
Generative AI technologies like ChatGPT and LLMs are becoming increasingly ubiquitous, but many businesses find that they can't fully use these innovations due to a lack of data governance.
Data governance is a concern for any company looking to adopt generative AI because data stored within an LLM, even a private LLM, can’t be easily governed the way you can govern access to tables in a database. And, unlike conventional applications, LLMs have no “delete” button.
There’s no straightforward mechanism to “unlearn” specific information, no equivalent to deleting a row in your database’s User table from an LLM. This is particularly worrisome because the “right to be forgotten” is included in privacy regulations like the EU’s GDPR and California’s CPRA.
In this post, we’ll explore what businesses want to achieve with generative AI, the privacy and compliance challenges they face, and the architectural solutions for meeting those challenges with data governance for generative AI. We will also show how using a zero trust data privacy vault simplifies data governance and frees companies to move generative AI from proof-of-concept to production.
What Businesses Want to Achieve with Generative AI
Businesses are eager to harness the power of generative AI for a variety of purposes, including:
- Content Creation: Generative AI can generate content, from articles to product descriptions, at a scale and speed that was previously unattainable
- Customer Support: Chatbots powered by generative AI can provide 24/7 customer support, improving customer satisfaction and reducing the workload on human customer support agents
- Data Analysis: AI algorithms can sift through vast amounts of data to identify trends, anomalies, and opportunities, aiding in data-driven decision-making
- Personalization: Generative AI can tailor recommendations and experiences for individual customers, enhancing user engagement and driving sales
- Research and Development: AI can assist in research, from drug discovery to scientific exploration, by analyzing complex data sets and generating hypotheses
These advancements hold great potential, but they come with a caveat – the need for robust data governance to prevent the exposure of sensitive data.
What is Data Governance?
Data governance is the overarching strategy and framework that controls who has access to sensitive data, for what purposes, for how long, and how it's stored. An effective governance strategy ensures data is handled consistently, securely, and in compliance with regulatory requirements – and it prevents sensitive data sprawl.
Data Governance: A Missing Feature for Generative AI
Without proper data governance, you can’t safely move past the proof-of-concept phase to harness the potential of LLMs without risking the exposure of sensitive data. And, exposing sensitive customer data like names and dates of birth, or company secrets as occurred at Samsung, can lead to privacy concerns from customers, reputational damage, and legal consequences.
The current moment with generative AI is somewhat similar to other historical periods when new innovations were introduced: everyone is thrilled to play with cutting-edge innovations, but we discover that these innovations are lacking some critical safety features.
For example:
- Early Cars Didn’t Include Seatbelts: In the early days of the automobile, seatbelts weren’t standard, and their absence posed significant risks to passengers
- Early App Stores Lacked Parental Controls: When app stores first launched, there was no effective way for parents to govern purchase controls for their children (resulting in lawsuits over in-app purchases)
Similarly, the lack of a built-in governance framework around generative AI hinders its adoption across critical business workloads.
The Importance of Data Governance
Data governance is not just about adhering to regulations; it's about ensuring that data is managed responsibly and efficiently. The key components of an effective data governance strategy include:
- Data Access Control: Specifying who can access data and under what circumstances
- Data Retention: Defining how long data should be retained and when it should be deleted
- Data Security: Implementing measures to protect data from unauthorized access or breaches
- Data Privacy: Safeguarding sensitive data and using it in compliance with privacy regulations
The Relevance of Data Governance for Generative AI
The relevance of data governance becomes increasingly evident when you consider factors like the following:
- Ongoing Governance Control: Data governance is not a one-time activity; it's an ongoing process to maintain control over data as new data sources and AI applications emerge.
- Privacy and Generative AI: The concerns related to data privacy and generative AI are multifaceted: beyond worries about including PII in training data, there's the challenge of protecting the privacy of inference data – files or text provided to LLMs by users
To illustrate the ongoing governance and privacy challenges businesses face, consider this hypothetical scenario: What if an employee enters personal information into an LLM prompt, quits their job, and then invokes the “right to be forgotten” (RTBF) under GDPR, CPRA, or a similar law?
It’s inefficient and very expensive to retrain a private LLM to make sure you delete this data, and with a public LLM you won’t have that option. To address these concerns and comply with an RTBF request, you need effective data governance to keep sensitive data out of LLMs.
Regulatory Frameworks and Compliance
If you handle sensitive personal data – names, social security numbers, etc. – you’re probably already familiar with compliance requirements related to this data. Data governance is a critical part of any regulatory compliance strategy. As AI applications proliferate, regulations surrounding data privacy and AI are continually evolving. Some notable regulatory frameworks include:
- GDPR (General Data Protection Regulation): GDPR mandates stringent data protection requirements for organizations that handle personal data, with severe penalties for non-compliance
- HIPAA (Health Insurance Portability and Accountability Act): HIPAA sets standards for the protection of medical information and patient privacy
- CCPA (California Consumer Privacy Act): This California state law gives consumers increased control over their personal data, and it was recently strengthened by the passage of CPRA (California Privacy Rights Act).
These are just the laws that are currently in effect. Currently, the EU AI Act is being finalized and stands poised to be the most impactful data privacy regulation since GDPR.
Resources like the IAPP’s Global AI Legislation Tracker can help keep you informed about the latest developments, and you can use resources from organizations like the FPF to define internal policies around AI use.
But, a better strategy is to proactively adopt data governance strategies that isolate, protect, and govern sensitive data so that when new regulations go into effect, you’re ready to comply more rapidly than your competitors.
Real-World Consequences of Mishandling Sensitive Data
Data mishandling can have far-reaching consequences, not only in terms of compliance but also on a company’s competitive position and reputation. Consider the Samsung ChatGPT misadventure, where source code was inadvertently leaked, exposing proprietary information and potentially damaging customer trust.
Strategies for Data Governance
Effective data governance involves a multifaceted approach to safeguard sensitive data while allowing organizations to leverage generative AI.
Here are some key strategies:
Data Minimization: The First Line of Defense
Data minimization is the practice of collecting and retaining only the data that is necessary for a specific purpose. By reducing the amount of sensitive personal data in circulation, organizations can significantly reduce the risk of data exposure, easing compliance with data privacy regulations.
Fine-Grained Access Control
Fine-grained access control provides individuals and applications with precisely the level of access needed to perform their tasks, preventing unauthorized access to sensitive data.
Data Anonymization and Pseudonymization
Anonymizing and pseudonymizing data are techniques that can help protect privacy while still leveraging data for AI training. By removing or obfuscating personally identifiable information before it reaches an LLM, organizations can prevent this data from being traced back to individuals, mitigating privacy risks.
Secure Data Sharing Mechanisms
Implementing secure data sharing mechanisms facilitates collaboration and data exchange with trusted third party services without compromising data privacy. Effective secure data sharing involves the use of encryption, access controls, and encrypted communication channels to share data with trusted partners while maintaining data security.
Implementing Data Governance for Generative AI
Generative AI introduces unique challenges to traditional data privacy models. To effectively implement data governance for generative AI, you need an architectural solution that keeps your sensitive data out of LLMs. This gives you the data governance you need to make effective AI governance achievable.
The Architectural Solution: Skyflow LLM Privacy Vault
Skyflow LLM Privacy Vault is specifically designed to provide comprehensive data privacy and data governance for generative AI applications. It lets companies realize the potential of AI while safeguarding sensitive data and easing compliance with privacy regulations.
Skyflow offers features such as fine-grained access control, data anonymization, and support for sharing sensitive data with trusted third party services. And crucially, it detects sensitive data and keeps it out of LLMs, without sacrificing the ability to later re-identify this data.
Skyflow addresses privacy concerns around LLM training (including multi-party model training) and inference from user inputs throughout each phase of the lifecycle of LLMs:
- Model Training: Skyflow enables privacy-safe model training by excluding sensitive data from datasets used in the model training process.
- Multi-party Model Training: Skyflow supports multi-party training so multiple parties can de-identify sensitive data and then build shared datasets that preserve data privacy.
- Inference: Skyflow also protects the privacy of sensitive data from being collected from prompts or user-provided files.
- Integrated Compute Environment: Skyflow LLM Privacy Vault seamlessly integrates into existing data infrastructure to add an effective layer of data protection that prevents plaintext sensitive data from flowing into LLMs, and only reveals sensitive data to authorized users as model outputs are shared with those users.
Skyflow LLM Privacy Vault de-identifies sensitive data through tokenization or masking and also lets businesses define terms that are too sensitive to feed into LLMs. It also supports data privacy compliance requirements, including data residency.
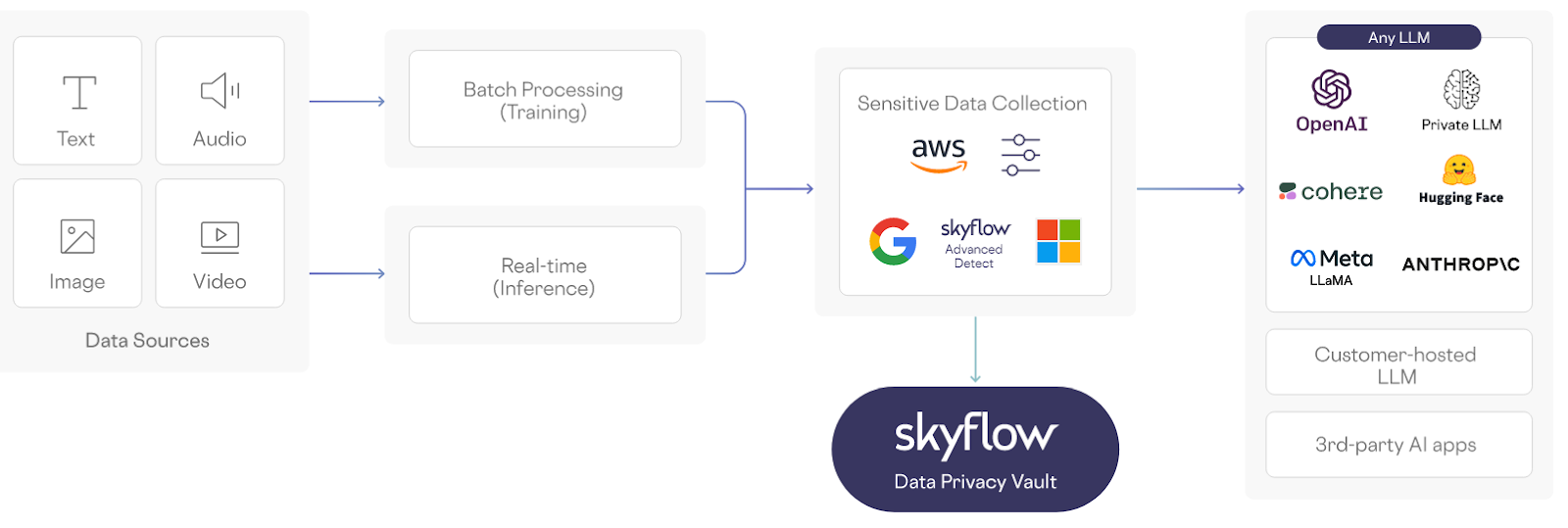
To learn more about how we protect sensitive data, check out our Skyflow LLM Privacy Vault blog post.
Conclusion
Data governance for AI is not just a necessity but a strategic advantage. A wide variety of corporate leaders, including Chief Privacy Officers, Data Protection Officers, CTOs, and CISOs play a crucial role in establishing and maintaining effective data governance practices. These practices are essential to help ensure that this technology is used responsibly and ethically.
As every company transforms into an AI company, it’s critically important to face data privacy challenges head-on. Without scalable AI data privacy solutions, businesses risk remaining stuck indefinitely in the “demo” or “proof-of-concept” phase.
By using Skyflow LLM Privacy Vault, companies can protect the privacy and security of sensitive data, protect user data privacy, and retain user trust when using LLM-based AI systems.